An Atypical Approach to AI in Education
Exploring the impact of AI on higher education institutions and assessment
In this interview with Dr. James Genone, we go through his experience in transitioning from educator to working at a university upstart and now at AI-startup Atypical.
James shares his views on the potential for AI to truly make positive change in our learning systems, the limitations of the chat interface, and the potential for conversational agents as a form of assessment - to uncover learner competence.
(The interview has been condensed and edited for brevity)
How did you get involved in education and AI?
I began my academic journey in philosophy and eventually moved into psychology. As my interests around the human condition evolved, I found myself drawn to cognitive science—an interdisciplinary field that bridges our understanding of the mind with computational theories and scientific research.
Exploring cognitive science led me to work on perception and perceptual learning, which are closely tied to the computational theory of mind. This theory suggests that if the mind functions like a computer, it might be eventually possible to build a machine with human-like capabilities.
I’m also a big science fiction buff, and books like Stephenson's "Diamond Age" inspired me to consider the potential impact of these developments on education. At the time, I quickly realized that most edtech tools used machine learning for basic tasks like classification without fundamentally enhancing teaching and learning.
These experiences revealed the limitations of existing approaches and fueled my desire for a deeper integration of AI in educational settings. This led to a big career shift—from teaching philosophy to developing curricula at Minerva.
The turning point came in the summer of 2022, when I encountered early large language models. The potential I saw in these technologies convinced me that a new era was dawning.
At Minerva, this pushed us to delve into LLM applications for assessments, and I spent considerable time experimenting with this emergent technology, driven by the belief that AI could truly revolutionize education and assessment practices.
Today, I’m at Atypical pursuing that exact mission - creating novel AI-enabled solutions for personalized tutoring, teaching, and the measurement of student success.
What are you spending your time thinking about most these days?
I've been spending a lot of time thinking about the right approach to adopting AI at scale. While there's plenty of enthusiasm and bold claims about how AI will revolutionize the world, there's also a significant amount of skepticism.
My view is that both extremes might be overstating the immediate impact. No matter how powerful a technology is, if it doesn't solve real, tangible problems, its impact will ultimately be minimal.
Historically, we've seen technologies in education, like MOOCs, scaled massively but with little benefit to actual learning. With AI, I'm focused on ensuring we avoid these pitfalls.
My primary concern is how we can best support teachers in their roles. Teaching is incredibly demanding, and I'm exploring ways to make educators' jobs easier and more effective. There is great potential - a lot of it dependent on behavioral change and support.
Despite the excitement, I'm still skeptical that AI has fundamentally changed the way we learn today. There are, however, innovative uses of AI outside of education that hold potential, which makes me think about what an AI-native education could look like.
Optimistically, I believe that industry will eventually mandate AI literacy, pushing higher education to adapt and possibly embrace practices we know are beneficial—like experiential learning, apprenticeships, and active learning. This pressure could create an opening for meaningful changes in how we educate, aligning academic training more closely with industry needs and future realities.
How should leaders at Higher Education institutions (HEI) think about leveraging AI?
Previously, my recommendations to HEI leaders largely centered on experimentation and exploration—a stance that remains relevant today as many have yet to adopt this approach.
However, leaders now need to think more strategically about how AI will impact the business of higher education. It’s going to become increasingly challenging for graduates to secure employment if they are not versed in the AI economy.
Non-elite institutions, in particular, need to reconsider the declining return on investment of a traditional university education if it does not equip learners to navigate the AI economy. This imperative extends even to trade schools; professions like electricians and construction workers will also experience shifts due to AI advancements.
The current structures of HEIs are becoming outdated. For instance, earning a degree solely in chemistry may be less beneficial than an interdisciplinary degree in science. This idea may sound reminiscent of approaches advocated by institutions like Minerva where I’ve worked, but AI's evolution has made the validity of these interdisciplinary and adaptive education models even more pertinent today.
In a recent interview, you noted that the chat interface was not the best one for education applications for AI. Can you say more about that?
Chat interfaces require users to know exactly how to guide the AI to help solve their problems, which can be a significant hurdle since not everyone is an expert - especially learners in a new subject.
Although chat interfaces are improving, they still tend to be generic and less effective unless the user's inquiries are very specific. As AI technologists, our challenge is to enhance the UX/UI to guide users more effectively—providing the right prompts and inputs to elicit the desired outputs.
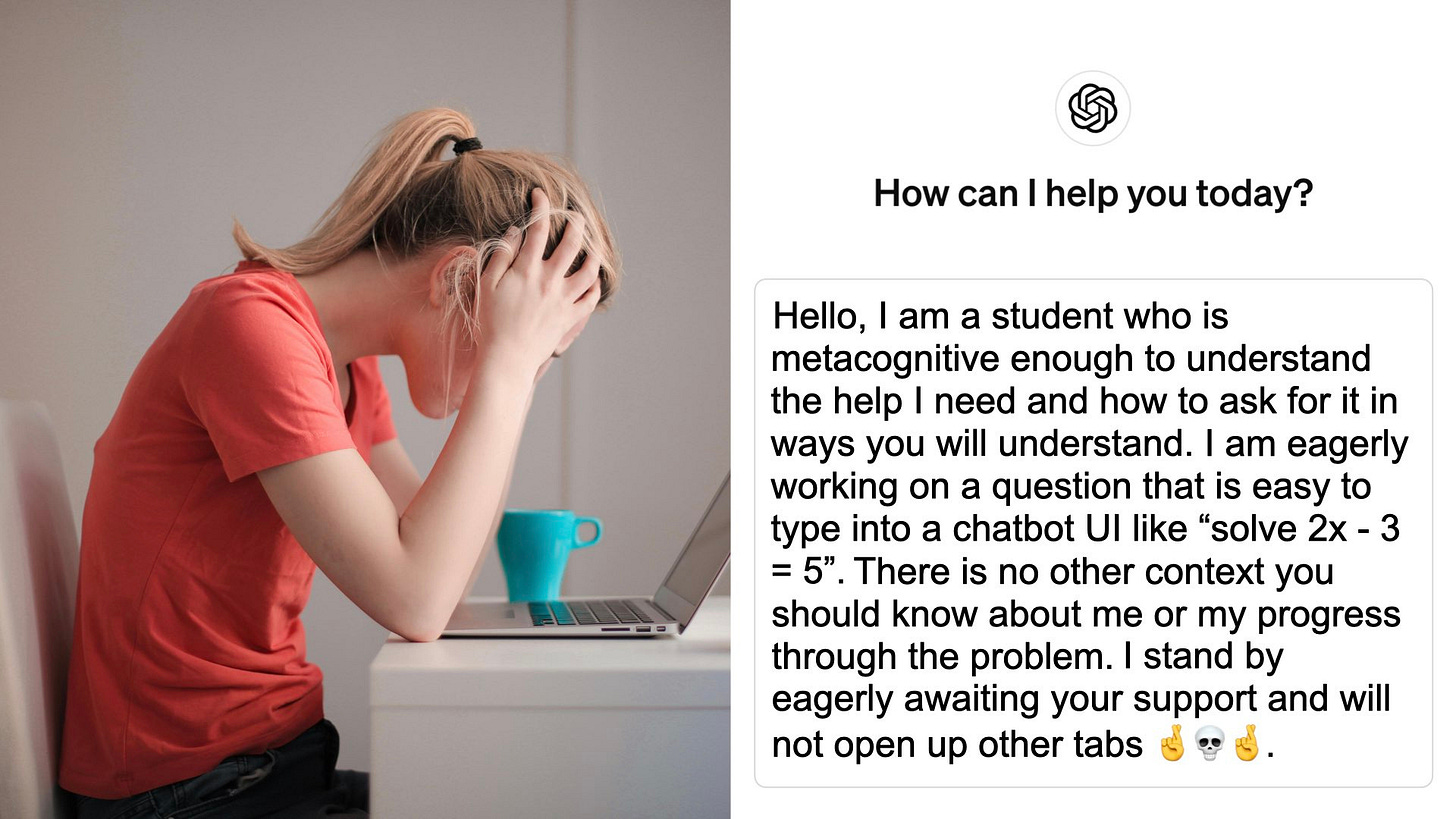
This task is not straightforward because it involves making assumptions, which could be incorrect. Moreover, we want to avoid overwhelming users with too many questions. It's a delicate balance to strike, but it's crucial for fostering good adoption of AI in educational contexts.
It's important to note that while AI models have become better at responding to well-crafted prompts, they haven't necessarily improved across the board.
How does the rise of AI affect the future of assessment?
As a teacher, I found that interviewing students about their work revealed much more about their understanding and thought processes than traditional exams.
This approach has also worked well in job interviews where you can get deeper insights into candidates' capabilities through discussions rather than standardized tests.
You essentially want to assess someone’s ability to perform real work and think about it. However, the challenge with these conversational assessments is that they are labor-intensive, typically requiring at least 45 minutes per person.
This is where AI, particularly advanced chatbots, could play a transformative role. They could effectively engage in these dialogues, assessing and adapting based on the student's responses, which would be especially potent if they could digest and analyze the student's previous work.
At my current company, we talk about using "tutor as an assignment," which benefits both students and teachers by mapping out areas of strength and weakness.
We are striving to build products that replicate high-quality teaching and assessment strategies using sound pedagogy. Our goal is to enable AI tutors to make strong pedagogical moves that help students get unstuck during their learning, particularly while doing homework.
I hope you enjoyed the last edition of Nafez’s Notes.
I’m constantly refining my personal thesis on innovation in learning and education. Please do reach out if you have any thoughts on learning - especially as it relates to my favorite problems.
If you are building a startup in the learning space and taking a pedagogy-first approach - I’d love to hear from you.
Finally, if you are new here you might also enjoy some of my most popular pieces:
The Gameboy instead of the Metaverse of Education - An attempt to emphasize the importance of modifying the learning process itself as opposed to the technology we are using.
Using First Principles to Push Past the Hype in Edtech - A call to ground all attempts at innovating in edtech in first principles and move beyond the hype
We knew it was broken. Now we might just have to fix it - An optimistic view on how generative AI will transform education by creating “lower floors and higher ceilings”.
I found Dr. James comment on MOOCs having little benefit in "actual learning" quite interesting. What is meant by "actual learning" and outcomes define good vs bad learning? For example, if I'm learning a new topic and I can understand/explain it conceptually but can't apply it practically (or vis versa), is that a good or bad outcome? We have a saying in my culture that translates to "memorizing with no understand" which I find stems from broken education systems as far high up as University level. What's meant by "actual learning" and how do measure this? I'm very new to the challenges in education/learning topic and trying to educate myself more about it. Would love more insight on this or resources that can help me understand more.